Our paper "The Other Side of Compression: Measuring Bias in Pruned Transformers" is accepted to IDA 2023
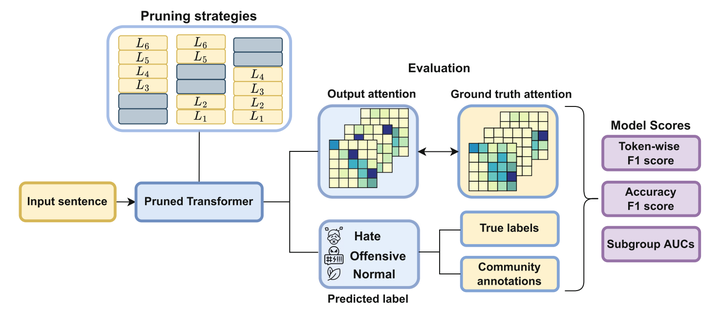
TL;DR We analyse the layers’ contribution to rational model decision-making in terms of performance and fairness.
Link for the paper : https://hal.science/hal-04104840/document.
The spread of offensive speech in social media is considered a precursor of numerous existing social issues, such as the distortion of victims’ portrayal in society, social tension, dissemination of entrenched stereotypes, provoking hostility and hate crime, not to mention the mental toll.Rational content moderation and filtering in social networks is the primary tool for preventing these consequences of offensive speech. Given the number of everyday social media posts, the need for automated content monitoring looks inevitable. Automated solutions also help to prevent moral damage and the negative impact of disturbing texts on annotators.Recently, algorithmic moderation has become a ubiquitous tool for the vast majority of social networks, including Facebook, YouTube and Twitter. Nevertheless, existing challenges of the hate speech detection task form a stumbling block to guaranteeing accurate and unbiased models’ predictions.
Context sensitivity and an unclear author’s intention are the main challenges at the data annotation stage. These factors are the primary sources of the annotators’ disagreement during the dataset creation. And the annotation bias in data influences learning bias accumulated when training a classifier, so the risk of the annotators’ bias inheritance increases. In the case of hate speech classification, there is a risk of unintended identity-based bias. For example, non-hateful texts containing mentions of gender, nationality or other protected attributes can be classified as a harmful utterances. The cases of biased decision-making are governed by law. For example, the social media platforms that signed the EU hate speech code have to delete posts using offensive and inappropriate language within 24 h.
Given the number of everyday posts to check, automated moderation system feedback delay is highly restricted. For that reason, accelerated and compressed models receive more attention for the task. Our paper presents one of the first attempts to analyze biased outcomes of compression in the context of hate and offensive language detection. In particular, we analyze the impact of encoder layer pruning in pre-trained Transformer Language Models (LMs, in short). Removing layers does not require additional fine-tuning and allows for explaining the contribution of the encoder blocks to model decision-making.
We analyse the layers’ contribution to rational model decision-making in terms of performance and fairness.
Contributions
- We measure identity-based bias in pruned Transformer LMs.
- We study which group of encoder layers (bottom, middle or upper) can be efficiently pruned without biased outcomes.
- We propose word-level supervision in pruned Transformer LMs as a debiasing method.